Data Analytics MSc students Stephanie Healy Fabrega and Indrajitrakuraj Ravi have been employed on a research project.
Date: 04 June 2021
Masters students at London Met have been working on a project to design a data ingestion pipeline.
The project, which was funded by the University, has been used to offer students opportunities to work on a research project to help them learn new skills. Stephanie Healy Fabrega and Indrajitrakuraj Ravi, two Data Analytics MSc students, have been recruited to the project as research assistants.
Data ingestion is the transportation of data from assorted sources to a storage medium, like a cloud, which can be accessed, used, and analysed by an organisation.
This pipeline can help empower London by saving researchers precious time for data analytics, support their decision making, and provide services and solutions for the city. This fits with London Met’s broader strategic aim to develop meaningful civic engagement between the University and the city, exemplified through the work of the London Met Lab, which launched last year.
Stephanie said: “Working towards building a data ingestion pipeline has been a great opportunity to grow not just personally but also professionally towards becoming a fully equipped data analyst. This research position has granted me the space for hands-on application of everything that I have learned in the Data Analytics MSc course, making my studies at London Met even more thorough and powerful.”
Indrajitrakuraj said: “The project itself is very interesting and I feel really proud and lucky to have an opportunity to work with Professor Qicheng Yu. This research has great potential to be helpful for students, researchers and even government agencies working on development as the project itself is about empowering London.”
So far, they have explored and identified more than 350 useful data sources in eight different catalogues involving aspects such as demographics and crimes to education and housing. Furthermore, they have created time and location dimensions within the data, covering both the last ten and the next ten years, which helps to create a meaningful foundation for the infrastructure of the research.
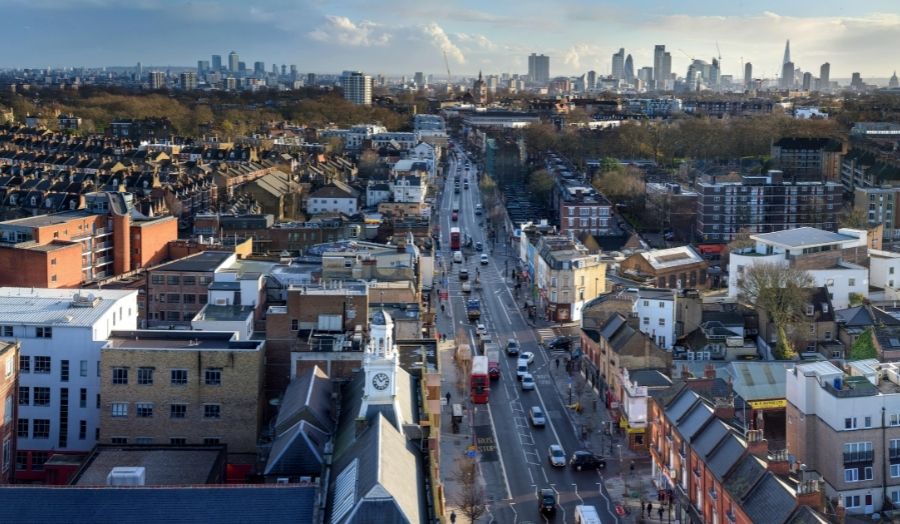